This piece was originally produced as part of my contribution to the Contemporary Issues in Ergonomics and Human Factors, a teaching module for the master's program at the School of Psychology, University of Derby. It was produced alongside a podcast to foster discussions with students during workshops and lectures
The driving style of automated vehicles is becoming an important factor for the automotive industry. It involves aspects such as the rate of acceleration and braking, the trajectory on bends, the smoothness or assertiveness of manoeuvres. Car manufacturers will have to program these behaviours into their automated systems.
But how should an automated vehicle behave? Myself and my co-authors asked this question to each other, and it became the title of one of our articles, listed at the end of this post (Oliveira et al., 2019). On closer examination, the article doesn’t give a clear answer. As academics like to do, it poses more questions. I think it’s because researchers, practitioners or policy makers still don’t know how to design and program these crucial details.
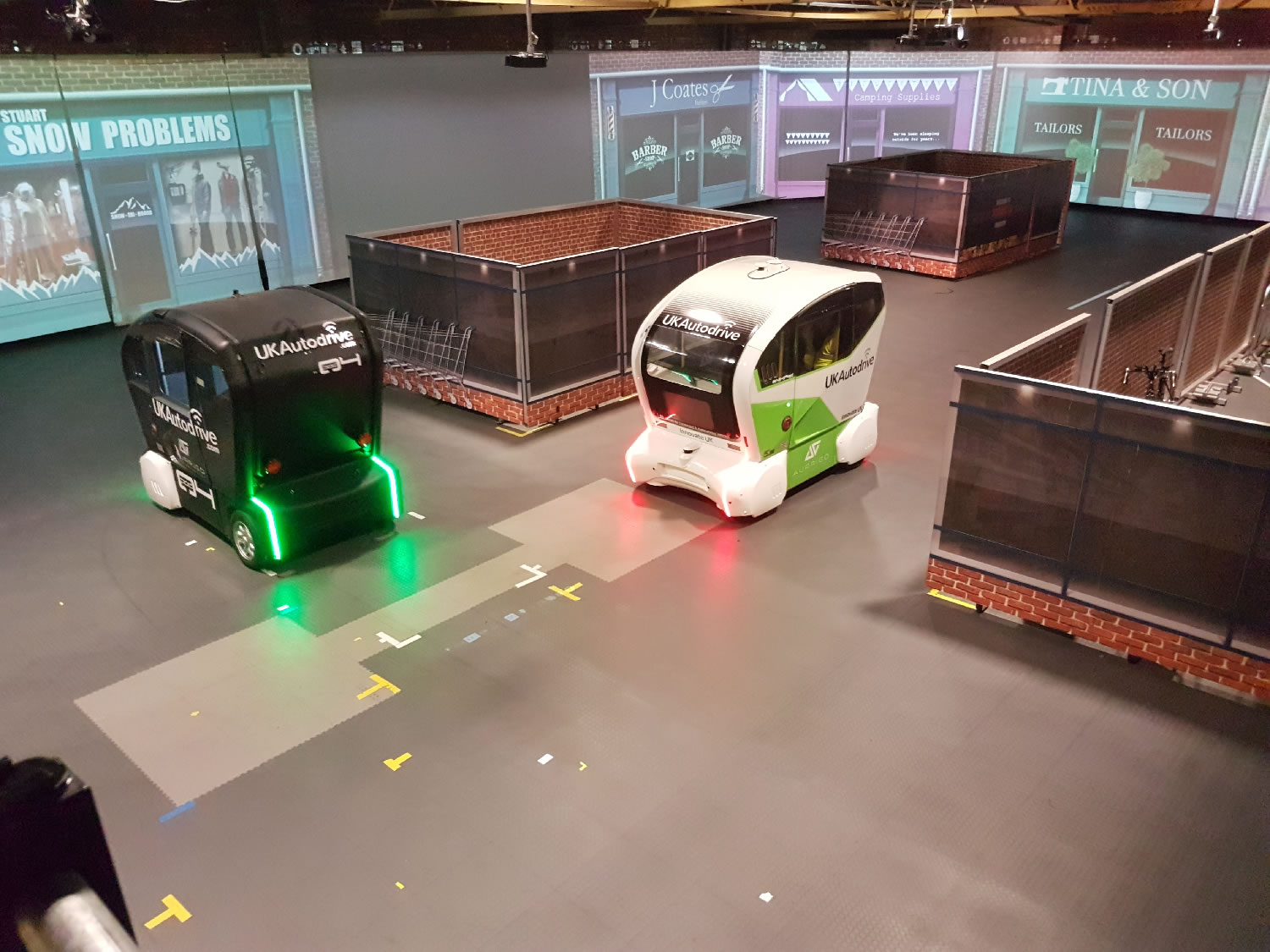
The idea for this article came in the summer of 2018, after we had run a series of studies measuring trust and acceptance of automated vehicles. We were working with Jaguar Land Rover and RDM Aurigo as part of the UK Autodrive project. We put participants inside those pods to test interfaces (Oliveira et al., 2020, 2018) or asked them to pretend to be pedestrians as the vehicles drove by (Burns et al., 2020, 2019). Figure 1 shows one of the highly autonomous vehicles that we used for our studies.
We always tried to combine quantitative measurements from questionnaires (for example trust, usability and motion sickness) with qualitative data from semi-structured, post-interaction interviews. This way, we could combine these datasets and provide a better picture of the reasons behind levels of trust and acceptance. I was the researcher assigned to perform the qualitative data collection and analysis, and often heard comments (usually complaints) about the vehicle’s driving style. Figure 2 shows comments from a participant, with the process of tagging statements into specific themes and giving recommendations for the design of these vehicles.
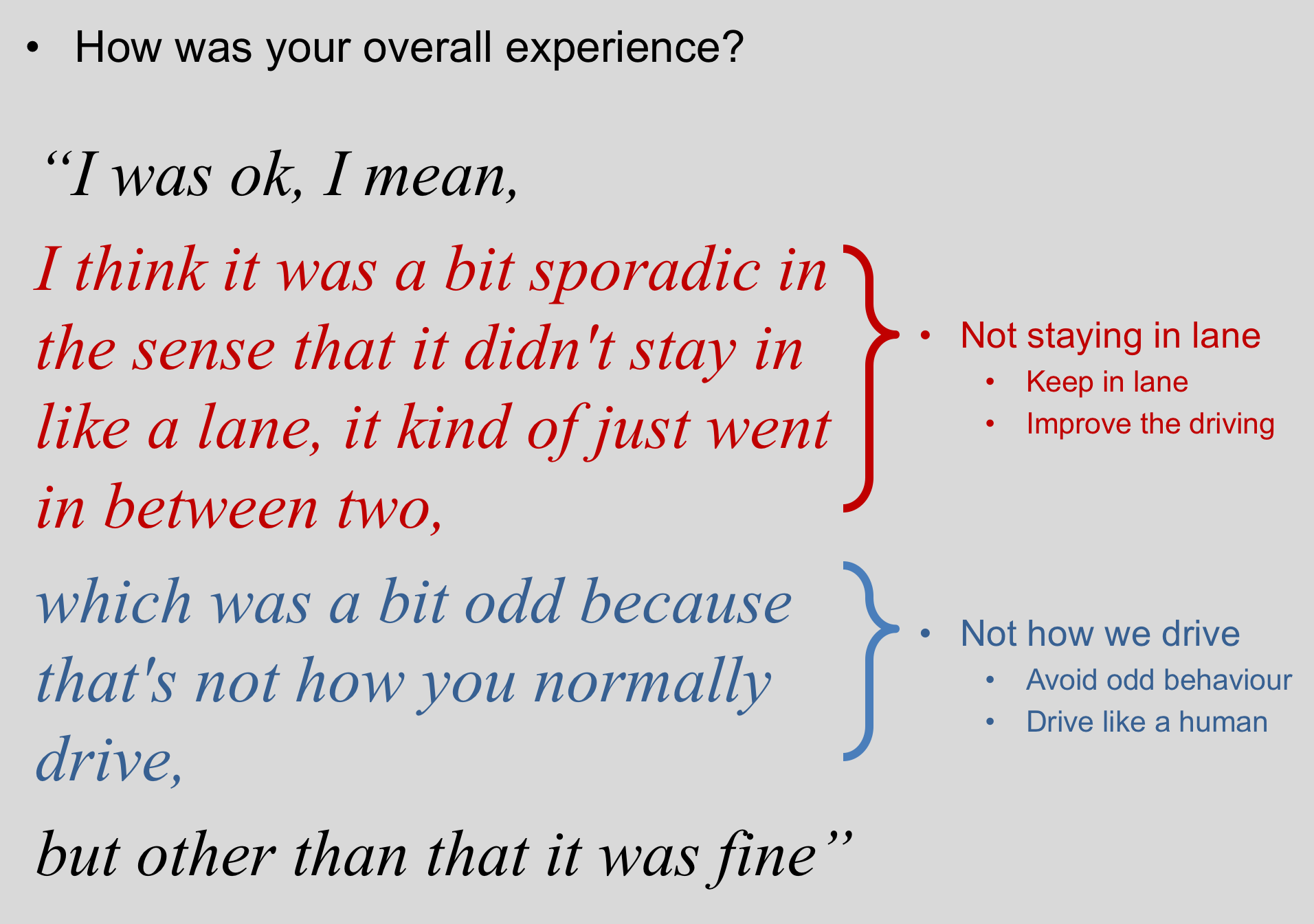
One complicating factor is that automated vehicles will ‘know’ more than occupants and take decisions based on information beyond the vehicle’s field of view (Qiu et al., 2017). The road infrastructure and vehicles will be able to collect information via cameras and sensors to share with other vehicles. For example, if an automated vehicle knows that the cross junction ahead is clear and there are no other vehicles coming on the other road, it could potentially just cross without stopping. Will we trust autonomous vehicles that drive assertively, or do we need them to drive like humans, as if they were looking before proceeding?
To try to answer these questions, we designed a study using two automated vehicles at the same time, negotiating junctions and corners automatically. Two different driving styles were presented to two groups of participants. The first was a vehicle programmed to drive similarly to a human, “peeking” when approaching road junctions as if it was looking before proceeding. The second design had a vehicle programmed to convey the impression that it was communicating with other vehicles and infrastructure and knew if the junction was clear so could proceed without ever stopping or slowing down. The opposite could also happen: a vehicle is aware of a hazard ahead and stops before it being clear to occupants, as illustrated in Figure 3.
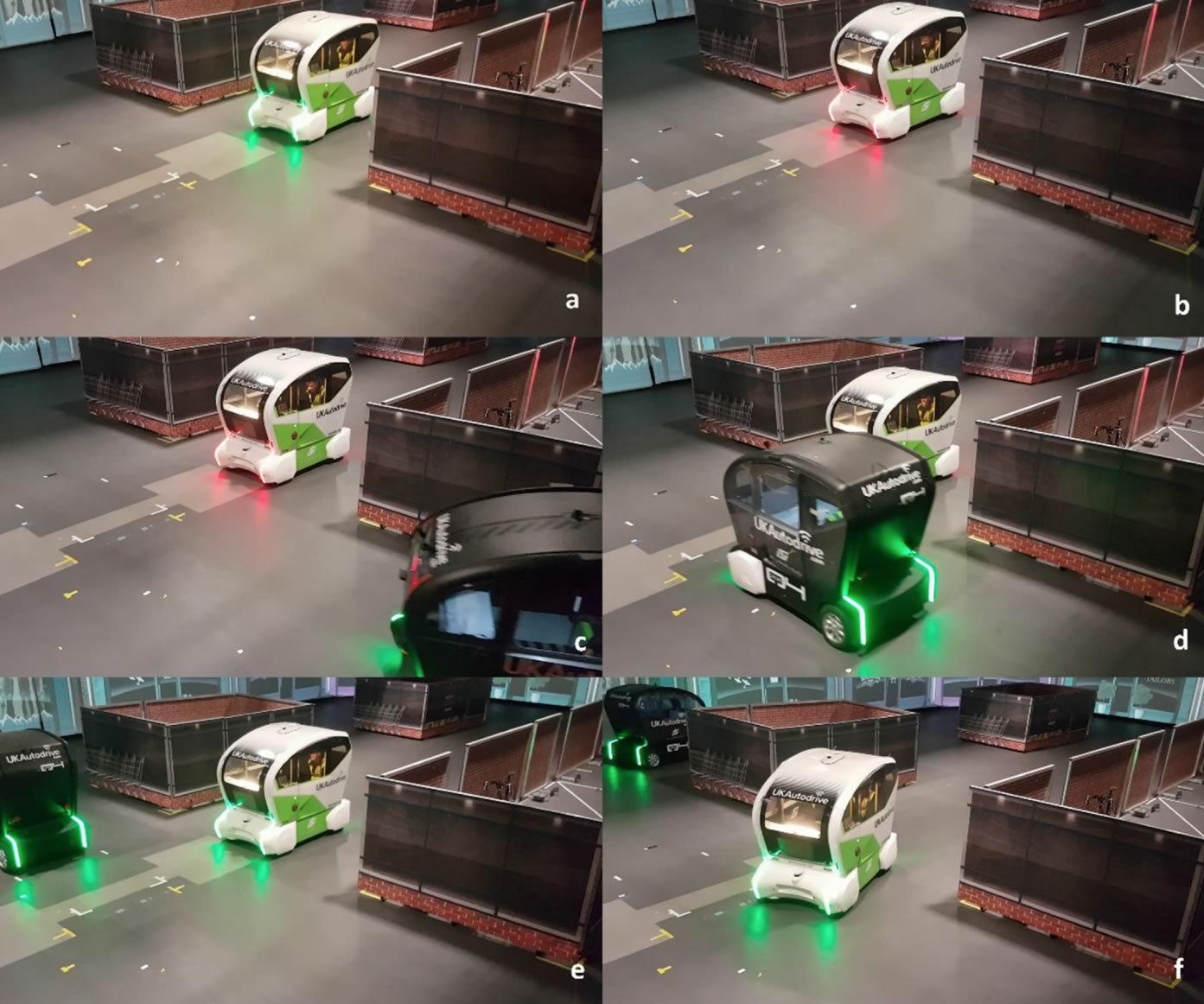
Results showed non-significant differences in trust between the two vehicle behaviours. However, there were significant increases in trust scores overall for both designs as the trials progressed. The post-interaction interviews indicated that there were pros and cons for both driving styles, and participants suggested which aspects of the driving styles could be improved. The paper presented recommendations for the design and programming of driving systems for autonomous vehicles, with the aim of improving their users’ trust and acceptance.
One debate that emerged from this study is the dichotomy between individuality and common good. The societal benefits of autonomous vehicles such as quicker interactions at junctions, fewer road collisions and less traffic will require some sort of orchestration of journeys via an overarching management system. Platoons of vehicles dispatching people and goods to different parts of cities in robotic precision seems out of a sci fi film, or from a military promotional demonstration video. But the personal freedom to decide the speed, route and driving style may disrupt this utopian autonomous transportation system. More research is needed to evaluate the acceptance of these driving styles in a broader sense. Or maybe once users better understand the driving systems and become familiar with the technology and the reasons behind its behaviour, they will be more trusting, accepting and likely to ‘let it do its job’.
This study generated large impact in the media. After writing the article, we contacted the department’s marketing & PR team, which wrote a news piece and sent to media outlets using their default channels. Within one week, our research was mentioned in more than 70 news portals and technology blogs. It was also translated into at least 5 languages.
References
Burns, C. G., Oliveira, L., Hung, V., Thomas, P., & Birrell, S. (2020). Pedestrian Attitudes to Shared-Space Interactions with Autonomous Vehicles – A Virtual Reality Study. In Advances in Intelligent Systems and Computing (Vol. 964, pp. 307–316). https://doi.org/10.1007/978-3-030-20503-4_29
Burns, C. G., Oliveira, L., Thomas, P., Iyer, S., & Birrell, S. (2019). Pedestrian Decision-Making Responses to External Human-Machine Interface Designs for Autonomous Vehicles. 2019 IEEE Intelligent Vehicles Symposium (IV), 70–75. https://doi.org/10.1109/IVS.2019.8814030
Oliveira, L., Burns, C. G., Luton, J., Iyer, S., & Birrell, S. (2020). The influence of system transparency on trust: Evaluating interfaces in a highly automated vehicle. Transportation Research Part F: Traffic Psychology and Behaviour, 72, 280–296. https://doi.org/10.1016/j.trf.2020.06.001
Oliveira, L., Luton, J., Iyer, S., Burns, C. G., Mouzakitis, A., Jennings, P., & Birrell, S. (2018). Evaluating How Interfaces Influence the User Interaction with Fully Autonomous Vehicles. Proceedings of the 10th International Conference on Automotive User Interfaces and Interactive Vehicular Applications, 320–331. https://doi.org/10.1145/3239060.3239065
Oliveira, L., Proctor, K., Burns, C. G., & Birrell, S. (2019). Driving Style: How Should an Automated Vehicle Behave? Information, 10(6), 219. https://doi.org/10.3390/info10060219
Qiu, H., Ahmad, F., Govindan, R., Gruteser, M., Bai, F., & Kar, G. (2017). Augmented Vehicular Reality: Enabling Extended Vision for Future Vehicles. Proceedings of the 18th International Workshop on Mobile Computing Systems and Applications – HotMobile ’17, 67–72. https://doi.org/10.1145/3032970.3032976